In the ever-evolving landscape of technology, a revolution is taking place right before our very eyes. This isn't just any revolution; it's one that's shaking up the sci-fi world and making it our reality: Artificial Intelligence (AI). Imagine a world where robots perform our everyday tasks, precision is no longer a human quality, and decisions are made at the speed of thought. Intriguing, isn't it? Well, let's not just dream; let's make it happen!
To conquer this fascinating world of AI implementation, we're offering you a map - five extraordinary strategies to unlock the golden treasure chest of AI. Get ready to ride the wave of this transformative tech and discover new territories of efficiency and profitability.
The Expanding Footprint of AI – Are You Ready for the Future?
AI is not just a technological marvel of our time; it's a revolution transforming industries across the globe. GlobeNewswire suggests the AI market is projected to reach a staggering $407 billion by 2027, experiencing substantial growth from its estimated $86.9 billion revenue in 2022. AI is expected to contribute a significant 21% net increase to the United States GDP by 2030, showcasing its impact on economic growth.
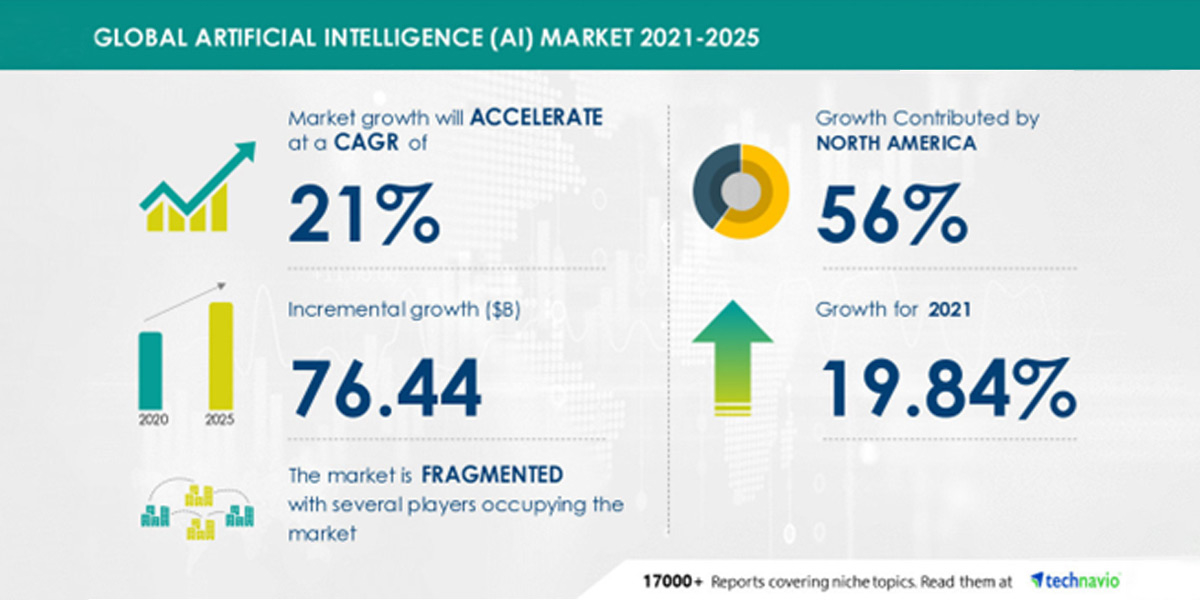
Tech giants like Microsoft, Adobe, and Alphabet have placed their bets on the AI table. For instance, Microsoft's multi-billion dollar dollar alliance with OpenAI aims to reimagine their Bing search engine, while Adobe has turbocharged its Experience Cloud offering by injecting it with AI and machine learning capabilities. Likewise, Google's parent company, Alphabet, has integrated AI and automation in nearly all aspects of operations.
In its magical prowess, AI is granting wishes to businesses brave enough to take the leap. However, unlocking this genie is no child's play. Fear not; the following steps will guide you on this enchanting digital transformation journey.
Step 1: Establishing Clear Project Goals and Guidelines
Establishing clear goals and guidelines is the first and foremost step in implementing an AI solution. This process involves identifying problems that can be resolved by applying AI and setting measurable goals. Understanding the organization's workflows, processes, and data can aid in identifying areas that can easily transition to AI.
Start with simpler, more achievable goals to gain familiarity with AI technology, then gradually move towards goals requiring a longer implementation schedule. The key steps involved in the first step would be.
- Identify the specific problems that AI can address in the organization.
- Set clear and measurable goals for the AI project.
- Understand the organization's workflows and data for successful implementation.
- Utilize relevant metrics and KPIs to measure concurrent improvements
- Gradually move towards more complex goals as your proficiency in AI increases.
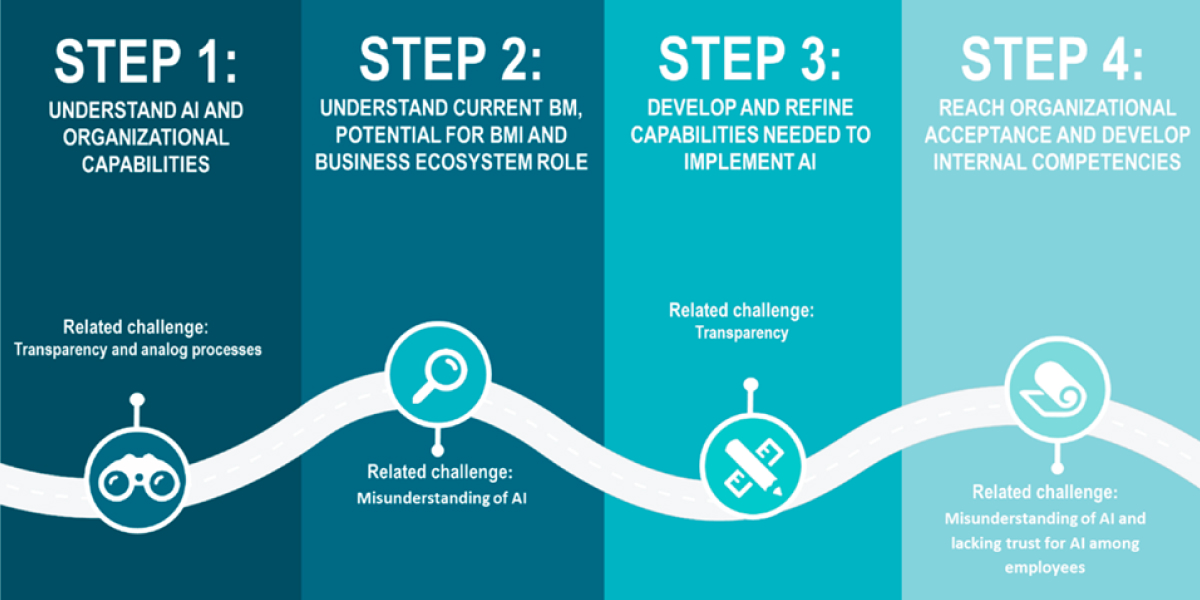
With a clear understanding of the goals in our minds, let's march forward to the next crucial component of our journey - ensuring we have the correct data at our disposal.
Step 2: Ensuring High-Quality Data Collection and Preparation
Data quality plays a significant role in the success of AI implementation. AI systems primarily depend on the quality of data they are trained on. Poor data can lead to inaccurate decisions by the AI system; hence, the data must be accurate, relevant, and consistent for reliable results.
Likewise, AI implementation can immensely contribute to business optimization by improving data quality and making it fit for advanced AI-powered analytics systems.
This improvement can lead to a myriad of benefits, such as cost reduction and enhanced decision-making capabilities. Detailed steps for ensuring high-quality data collection and preparation include:
- Appraising existing tools to ensure they can successfully develop, run, and maintain AI software and have the capabilities to prepare data for training the algorithm.
- If necessary, Assess the data quality and take steps to improve it, using data cleaning and preprocessing techniques to eliminate errors, inconsistencies, and duplicate records.
- Ensuring that the data is representative of the real-world scenario that the AI model is trying to predict. Businesses may need to generate synthetic data sourcesif there is not enough data.
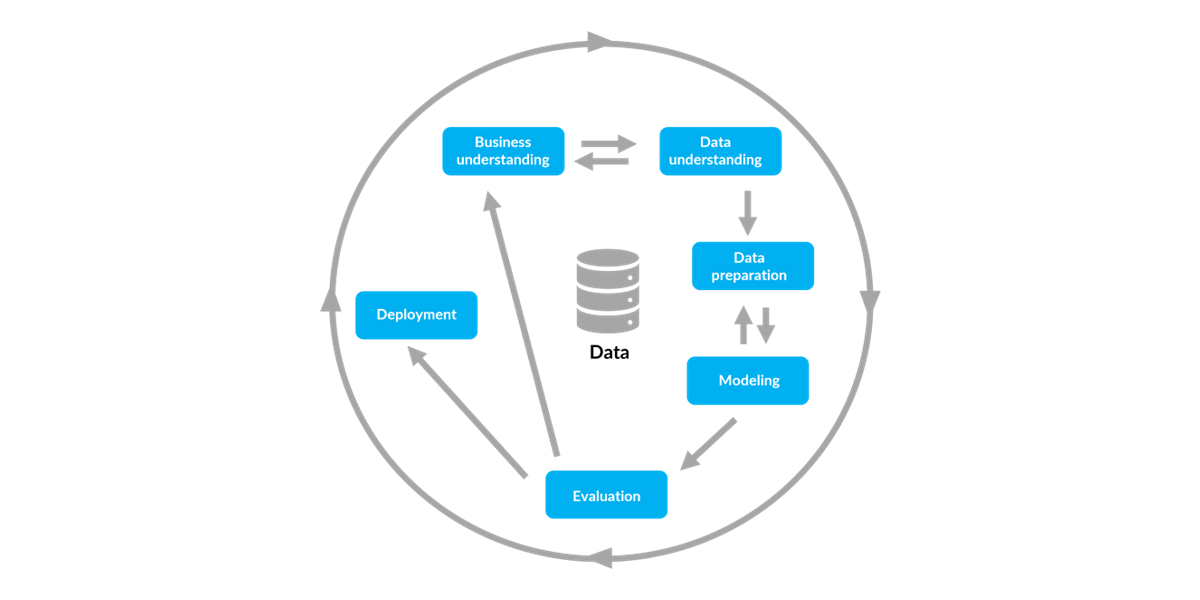
Armed with quality data, our AI journey brings us to the next checkpoint - assembling a formidable team of professionals and selecting the perfect model that aligns with our goals.
Step 3: Assembling the Right AI Team and Choosing an Effective Model
A successful AI implementation strategy requires the correct data, a competent team, and an appropriate model. Building the right team, for example, can start with hiring a data scientist who can handle a range of tasks from data analysis and visualization to developing cutting-edge AI models.
These professionals are pivotal in preparing and merging datasets, crafting relevant attributes for feature engineering, and deploying models for integration into the workflow. Key responsibilities of a data science team include.
Data Preparation & Merging Merging datasets into a single source and making them AI-ready.
Data Visualizations Creating charts and visualizations to help answer pertinent questions.
Feature Engineering Using domain knowledge to craft the right attributes to bring you closer to your goal.
Machine Learning Models Developing and implementing models for various use cases, including classification, regression, time series, and clustering.
Foundation Models Assisting in the utilization of modern breakthroughs like GPT-4.
Model Deployment Deploying models and helping to integrate them into the workflow, end-to-end.
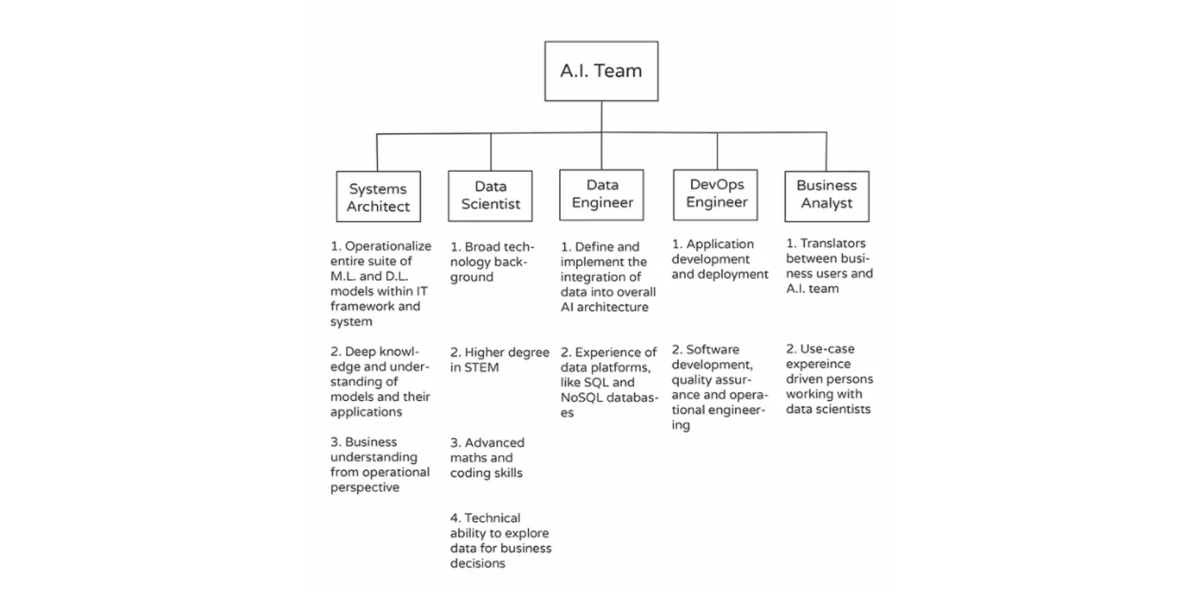
With the right team and model on our side, we're ready to dip our toes in the water. It's time to put theory into practice with pilot projects.
Step 4: Test the Waters with Pilot Projects
After setting your business goals, compiling quality data, and onboarding the right AI teams, it's time for pilot projects. Starting small with pilot projects is a prudent approach to AI integration. This method allows organizations to test the waters, validate the efficiency of AI models, and estimate the potential ROI without disrupting existing systems on a large scale.
Pilot projects provide the opportunity to learn, adapt, and improve AI models based on real-world experience, which can lead to better outcomes when scaling up. Following are some critical steps for initiating AI integration with pilot projects:
Identify a Suitable Use Case: The first step is to identify a use case that can benefit from AI and is feasible to implement on a small scale. The chosen use case should have measurable outcomes and align with the organization's strategic goals.
Assemble a Cross-Functional Team: A team composed of individuals from different departments can provide diverse perspectives, fostering creativity and ensuring the chosen AI solution meets various needs across the organization.
Develop or Procure the AI Model: Depending on the organization's capabilities and resources, this might involve developing an AI model in-house or procuring one from an external provider.
Implement and Monitor: After the AI model is ready, it is integrated into the existing system for the chosen use case. It's crucial to closely monitor the model's performance to identify any issues and make necessary adjustments.
Evaluate and Learn: Post-implementation, the team should evaluate the project's success based on predefined metrics. Insights gained from this evaluation can be used to improve future AI implementations.
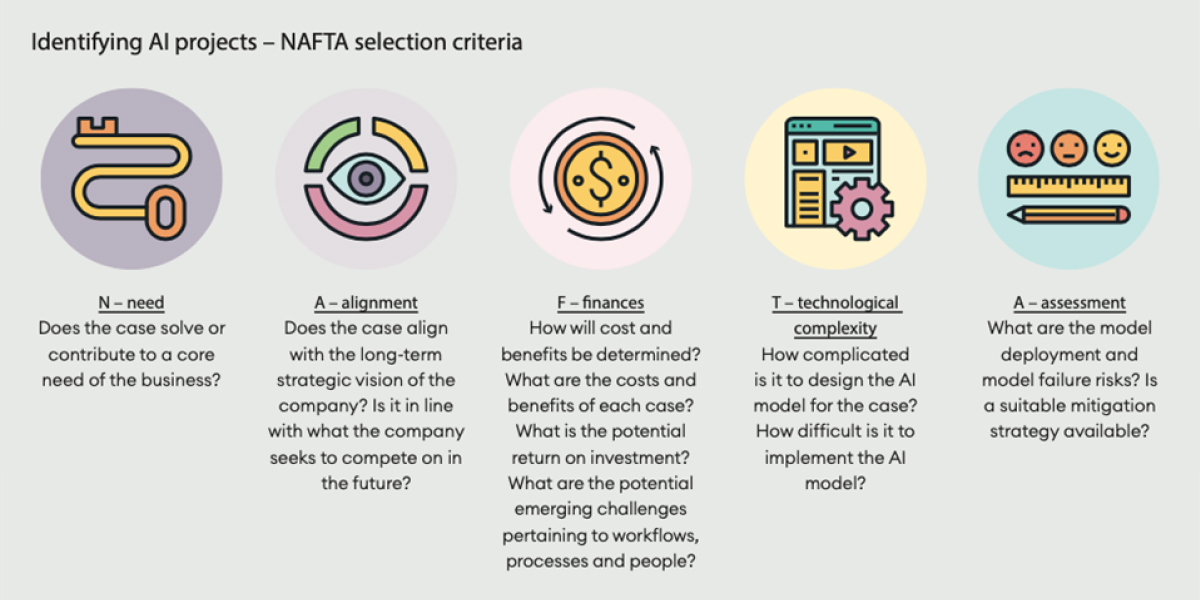
Fresh from the learning curve of our pilot projects, we must now tackle an often-overlooked but vital aspect of AI implementation – the ethical considerations.
Step 5: Ethical Considerations in AI Implementation
No AI implementation strategy can be complete without emphasizing and strategizing ethical considerations. These refer to the guidelines and principles that govern the use of AI in a way that respects human rights and values, maintains privacy and security, and ensures fairness and transparency.
It is not enough to have technically sound AI systems; they also need to be ethically sound to gain the trust of the people who will use them and be affected by them. Key ethical considerations include:
Transparency: AI systems should be transparent in their operations and decision-making processes.
Fairness: AI systems should not perpetuate or exacerbate existing biases and inequalities.
Privacy and Security: AI systems should respect individuals' privacy and ensure their data's security.
Accountability: There should be mechanisms in place for holding AI systems and their operators accountable for any harm they cause.
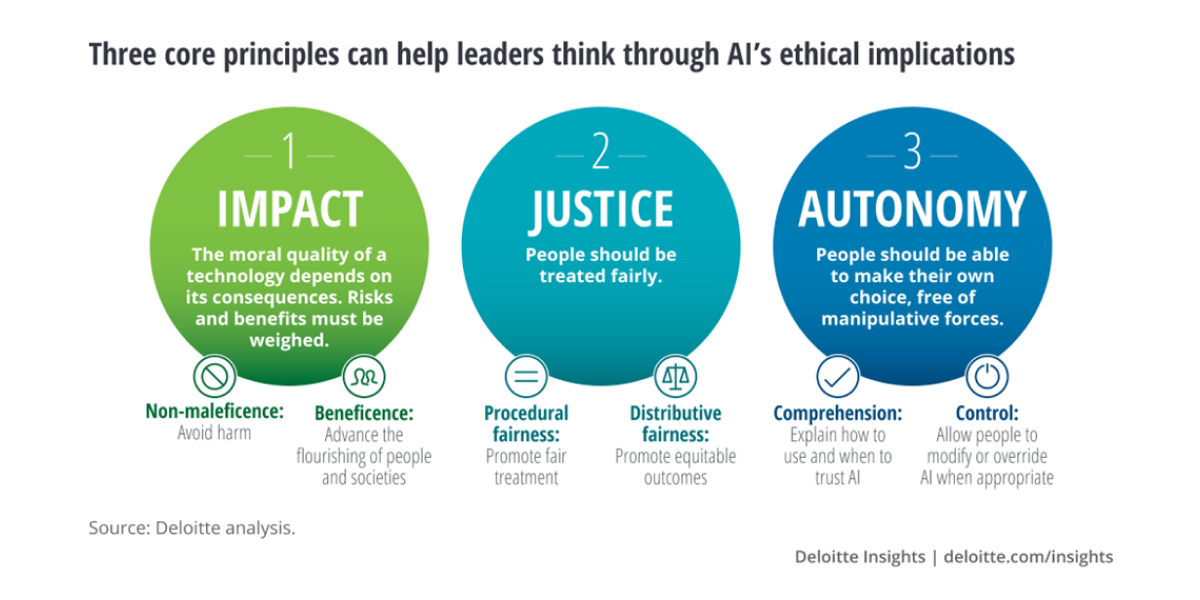
Final Words
The future is knocking at our door, and AI holds the key. The question is: are you ready to step into a new era of innovation and transformation? The path may seem complex, but by adopting a strategic implementation approach, you can unleash the enormous potential of AI and drive your business to uncharted territories of optimization and profitability. Want to ride the AI wave but feel like you're in over your head? Aspired is here to help you bring your AI vision to life with an armory of seasoned AI engineers and data scientists. Don't wait; dive into the future with Aspired Now!